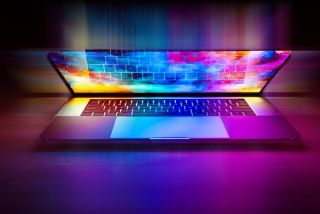
Source: Joshua Woroniecki / Pixabay.
There’s a new “chicken or the egg” question that’s gaining traction in large language models (LLMs) like ChatGPT: Is it the model or the method that drives optimal results?
This question often arises in the medical sector, where specialized training is considered to be essential. A recent study centered around GPT-4, well-established for its versatile approach, sheds light on this debate. The study revealed that strategic prompting may not only compete with but potentially outshine traditional model training in medical contexts.
A Shift in Approach: The Rise of Prompting Techniques
Traditionally, the prowess of LLMs in specialized areas such as medicine has been linked to intensive, domain-specific training. Yet, the latest findings introduce a surprising shift. The study suggests that GPT-4, despite being a generalist model, can reach remarkable heights in medical tasks through astute prompting, a realm previously dominated by specialized models.
Medprompt: A Case Study in Enhanced Medical AI
At the heart of this fundamental shift is the Medprompt study, which delves into GPT-4’s medical capabilities without extra training. Medprompt employs three groundbreaking techniques:
- Dynamic Few-shot Selection: This approach tweaks the few-shot learning method to pick examples closely related to the language of the task at hand, enhancing the model’s contextual response accuracy.
- Self-Generated Chain of Thought (CoT): GPT-4 is encouraged to autonomously produce detailed, step-by-step reasoning, leading to responses that align more closely with its processing strengths.
- Choice Shuffle Ensembling: In multiple-choice settings, this technique rearranges answer choices to combat bias, ensuring responses are content-driven.
Data Results: A Testament to Medprompt’s Efficacy
The Medprompt method significantly outperformed state-of-the-art specialist models like Med-PaLM 2, achieving a 27 percent reduction in error rate on the MedQA dataset (USMLE exam). Remarkably, it surpassed a 90 percent score threshold, a first in this domain. These results underscore the efficiency and accuracy that smart prompting brings to medical LLMs, challenging the necessity of extensive model training.
Transforming Medical LLMs Through Prompting
This advancement in prompting methodology holds significant implications for medical LLMs. These models typically require extensive training on specialized datasets to accurately address complex medical inquiries. Medprompt challenges this standard, demonstrating that a generalist model, with skillfully crafted prompts, can achieve comparable or even superior outcomes.
The Benefits of Prompting
- Flexibility: Unlike fixed training, prompting allows for adaptable adjustments tailored to specific tasks.
- Efficiency: Training on new data demands substantial resources. Prompting offers a leaner, more efficient alternative.
- Wide Applicability: Beyond the confines of medicine, these prompting techniques can be adapted to a multitude of fields.
Practical Application of Medprompt Strategies
While the Medprompt study focuses on medical LLMs, its principles are broadly applicable to the everyday use of GPT-4. The emerging reality is that prompt engineering offers fascinating opportunities to “focus” the discussion into a silo of expertise that provides an intellectual underpinning for the response.
- Contextualized Prompts: Align your prompts closely with your query, providing clear context for the model.
- Encourage Elaborate Reasoning: Prompt GPT-4 to detail its answers, guiding it to unfold its reasoning process.
- Combat Bias in Responses: In multiple-choice scenarios, shuffle answer options in your prompts to ensure consistency and unbiased responses.
The Medprompt study not only reshapes our understanding of LLMs in specialized sectors like medicine but also highlights the efficacy of intelligent prompting as a viable alternative to extensive model training. These insights lay the groundwork for more effective and efficient utilization of LLMs in various domains, broadening their impact in both specialized and everyday applications. Simply put, the power is often in the prompt, And it’s critical that we understand the “dialogue” that we leverage with LLMs to drive optimal results.
+ There are no comments
Add yours