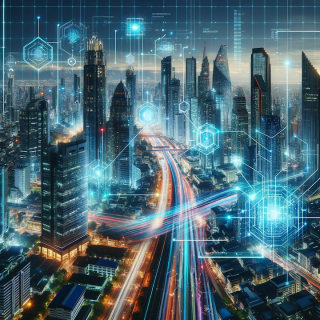
Source: Art: DALL-E/OpenAI
Often, Large Language Models (LLMs) like GPT are oversimplified as mere predictors of the next word in a sentence. This view, however, scarcely scratches the surface of their intricate design and potential. From my perspective, LLMs are akin to digital cartographers, distilling a vast expanse of human knowledge and experience into a dynamic, accessible map of our cognitive landscape. Let’s look beyond the myth of simple word prediction, to appreciate the true essence and potential of LLMs.
The Intricate Fabric of Large Language Models
LLMs are not mere word predictors; they are mirrors reflecting the intricate patterns of our world. They assimilate a colossal corpus of text, ranging from literary works to scientific discourses and daily conversations. From this amalgamation, they forge a representation of human cognition and language. This process is analogous to cartography. Just as a map presents a simplified version of physical geography, an LLM offers a condensed, navigable portrayal of human knowledge and discourse.
Crafting the Digital Atlas
The odyssey of an LLM begins with pre-training, where it grasps the rudiments of language and starts to piece together a preliminary worldview. This stage resembles the initial drafting of a map’s contours. As LLMs evolve, their pre-training grows more sophisticated, enabling a richer and more nuanced comprehension of the data, akin to refining a rudimentary sketch into a detailed, high-resolution map.
The Art of Fine-Tuning and Human Interaction
Post pre-training, LLMs undergo a critical phase of fine-tuning and human reinforcement, a transformative period that molds them into specialized instruments adept at understanding specific contexts and nuances. This stage, called Human Reinforcement Learning from Human Feedback (RLHF), is like customizing a general map for specific, intricate uses.
Human reinforcement is pivotal in this phase. Feedback from a diverse range of users, from experts to everyday individuals, directs the model’s learning, aiding in error correction and response refinement. This interaction is reciprocal; as humans educate the model, they also glean new insights from it. This cycle of learning is perpetual, enabling LLMs to adapt to evolving languages, trends, and knowledge domains. It’s akin to perpetually updating a map with fresh information to maintain its relevance and accuracy.
Additionally, human reinforcement imparts LLMs with an understanding of language nuances and context. Through varied interactions, these models learn to respond not just accurately, but also with appropriateness, acknowledging the complexities of human communication. This aspect elevates LLMs to invaluable assets in our digital ecosystem.
Navigating the Cognitive Seas
In an era brimming with information, LLMs stand as beacons, guiding us through this data deluge. They are far more than tools for information retrieval; they are co-explorers, enhancing our comprehension and interaction with the world in innovative ways.
As we advance, LLMs are increasingly becoming cognitive companions, assisting us in deciphering the vast, intricate tapestry of human knowledge. They are not just technological marvels but catalysts for intellectual discovery, urging us to reevaluate our perceptions of knowledge, creativity, and the potential of AI. Engaging with these models is imperative for educators, leaders, and learners as we chart our course through the complex terrains of the 21st century. This journey with LLMs symbolizes our unyielding quest for understanding and innovation, challenging us to harness their transformative power responsibly as we continue to unravel the mysteries of the human mind and the digital universe.
+ There are no comments
Add yours